(Article au format PDF)
We propose a model to analyse the vocabulary of a
corpus. This vocabulary is divided into two groups. First, the author uses
the same general words whatever the circumtances. Second, several
specialised vocabularies are used in only one part of the corpus. General
words may appear everywhere in the text : their increase with the
corpus' size can be estimated with Muller's formula. On the contrary,
specialised vocabularies grow proportionally according to the corpus' size.
We calculate the relative importance of the two vocabularies. This calculus
gives an estimation of the lexical 'specialisation' in the text.
The 'vocabulary partition model' is easy to compute and
it works well. It has been applied to several corpora to locate stylistic
or thematic changes and to measure the vocabulary richness with three
indicators : diversity, specialisation, oddness.
Vocabulary richness is often used in quantitative
stylistics. It measures the number V of different words in the N tokens
used in a text (we use the terminology and symbols proposed by Charles
Muller, 1977). One researches the ideal "norm" and unique
indicator, valid in any cases whatever length or nature of analysed
texts, but none of these formulas appears satisfactory. So one now compares
directly some texts when their lengths are similar
or by shortening them to the size of the most little one. (Muller, 1977 et
1979b ; Bernet, 1988).
Firstly, this paper shows how the analyse must take into
account the "specialisation" of the vocabulary. Then, we propose a division
of the "vocabulary richness" according to three notions (diversity,
originality and vocabulary growth ).
Vocabulary Spécialisation
Muller propose to calculate V' number of
different words expected in an excerpt of N' types, with
N'<N by this way : the V words, in the whole text, are
graded in order of frequency in n classes of frequency. Define
Vi as the number of words which have the i frequency,
V' is approximated by this formula :
(1) V'(u) = V - with Qi(u) = (1 - u)i and u =
This "Muller formula" has two limits :
Fn < N' < (N-Fn) in which Fn is the highest frequency
in the text.
Between these limits, one can calculate numerous
values and, with these values, draw a theoritical curve of "vocabulary
growth" (Muller, 1979a and Hubert-Labbé, 1988a). In the figure 1,
one can see the observed and calculated values on the press conference text
by Général de Gaulle on January the 31th of 1964.
In this experiment, the calculated values are always
under the observed values : it is a very usual characteristic which
Muller noted and explained by "vocabulary specialisation" in parts of
texts. According to Muller, the highest this specialisation is, the
greatest is the difference between calculated and observed values (Muller,
1977, p. 142-144). In consequence, the vocabulary specialisation can be
measured by the difference between the real curve of vocabulary growth and
the Muller's curve. Define p this specialisation, the figure 2 presents
shapes of vocabulary growth.
Fig. 1. Observed and Calculated Values on a Press
Conference by Général de Gaulle (using Muller's Formula).
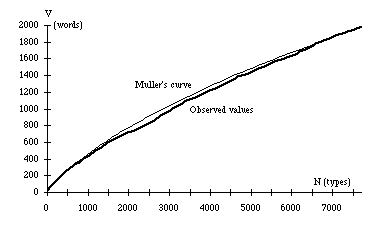
Fig. 2. Theoritical Shapes of Vocabulary Growth (P =
Vocabulary Specialisation).
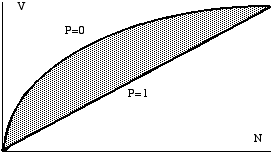
Nearly all the possible values are included in the
figure 2 shaded area. In case of no specialisation, the observed curve
coincides with the Muller's one (formula 1). On the contrary, if p is equal
to one, all the words are specialised and V' is then a linear function of
N' :
V'p=1(u) = u .V
Between these two extreme cases, the mathematical
expectation of the number of different words, in an excerpt of N' words,
is :
(2) V' (u) = p.u.V + q [ V - ] with q = 1 - p
The coefficients p and q measure the relative size of
two parts of text vocabulary : the first one contains the p.V
specialised words ; the second part contains the q.V words whose
probability of appearing at some stage in the text is constant. For this
reason, we propose to name p "coefficient of vocabulary partition"
(Hubert-Labbé, 1988b). In the formula (2), the first term estimates
the weight of the specialized vocabulary and the second, the general
vocabulary (with Muller's formula).
Practically, coefficient p is calculated this way :
the text is divided in a certain number of excerpts and, at each caesura,
the differents words are counted since the beginning of the text. The
lenghts of these excerpts are not necessarily equal and the caesura can be
placed everywhere. For K excerpts, the value of p is that which minimises
the sum of the quadratic deviations between the observed values
V'*(uk) and the calculated values (V' (uk)
). We obtain :
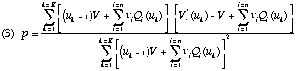
Formula (2) and (3) are easy to compute. Of course, the
accuracy of results is influenced by number and quality of observations. It
appears that no less than ten values of V'*(uk) are
necessary, evenly distributed in the texts or corpus. Given this cautious,
numerous experiments proved that p is actually independant of size and
number of excerpts. The figure 3 presents our findings on the same text by
General de Gaulle : theoritical curve now coincides well with observed
growth of vocabulary.
Fig. 3. Observed and Calculated Values on a Press
Conference by Général de Gaulle (using the Coefficient of
Vocabulary Partition)
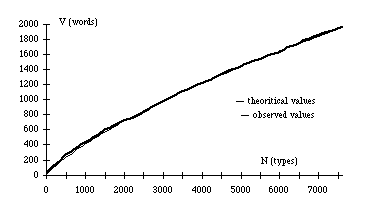
With this formula, one can compare, without error, the
"vocabulary richness" of several texts by shortening them to the size of
the little one, following the way proposed by Muller. At the end of this
presentation, a table gives results of this comparison between press
conferences by President de Gaulle (between 1958 and 1969). Two values of
V' are calculated with N' = 5299 types (size of the shortest
conference). The first one is obtained with "Muller's formula" (1) and the
second with "partition formula" (2) : Muller's formula can deeply
overestimate the vocabulary richness of the longest text when his
vocabulary has a high specialization (which is the case of conferences
holded on January 1964 and October 1966).
Specialization, Diversity, Originality and Vocabulary
Growth
Since "vocabulary richness" is a complex phenomenon, we
propose to divide this concept into four simple notions : diversity,
specialization, originality et vocabulary growth.
1. The vocabulary specialization is calculated
with p (partition coefficient). It underlines the author's ability to adapt
his vocabulary to the themes or, on the contrary, his propensity to use the
same words whatever the subject is. Practically, we never met texts in
which specialization overcomes 0.45. Above 0.3, the specialization is high
(it can also be interpreted as heterogeneousness
) ; between 0.29
and 0.15, the specialization is average ; under 0.15, it is low. The
values near or equal to zero are not unusual. Sometimes, in short texts,
some observed values can go above the Muller's curve, indicating a kind of
"hyper-generality".
At the end of this presentation, a table shows the
values of the partition coefficients in the General de Gaulle press
conferences. Usually, when he prepared his speeches, de Gaulle used a low
specialized vocabulary. On the contrary, during the December 1965 campaign
for presidential election, he had to improvise three T.V. interviews :
specialization was high : 0.34 in average. In other terms, the
"generality" of his speaches was a deliberate characteristic.
2. The vocabulary diversity indicates the
author's propensity to diversify his words or to avoid
repetition. The indicator of vocabulary diversity is the number of words in
the (N-1) excerpts of N' types which can be divided in a text. The
indicator of diversity is calculated from formula (2), selecting one value
of N' in order to compare texts or excerpts of different sizes (we choose
1.000 types).
Using "natural" caesuras, as chapter or plays, we can
study the text structure which is not taken into account by the Muller's
method. For example, the press conferencies of Général de
Gaulle have around 411-481 words for 1.000 types (see Table in annexe).
These values caracterize well-written texts (in improvised interviews of
December 1965, the indicator is less than 400). In other words, General de
Gaulle wrote his press conferencies and learnt them by heart ! It is
interesting to see that highest diversity is reached in critical times
(declaration about "autodétermination" of Algeria in autumn 1959,
presidential election of 1965, iniatives after March 1967 parliamentary
election or after May 1968).
A low diversity shows a poor preparation or a pedagogic
intention (by underlining the main points). On the other side, a high
diversity shows the intention to avoid monotony or a polemic will. But,
this intention of diversity can cause a low specialization ; so, a
combination of high diversity and good specialization reveals a very well
prepared text : it is the case of conference held on September 1965
which is also the longest (General de Gaulle prepared his second
candidature for presidency)
3. The vocabulary originality measures the
difference between vocabulary of an excerpt and the one of the whole text.
For this calculation, one can use the vocabulary specificities (Lafon,
1984). But specific words densities are partly dependent of excerpts' sizes
so that it is not possible to compare texts of different sizes. We propose
a less sophisticated way by using "proper vocabulary" (i.e. words used only
in a single excerpt). The more numerous is this proper vocabulary, the most
original is the text. Like specialised vocabulary, proper vocabulary is
dependent of excerpt size. Define VP the number of proper words found in
the K excerpts in the text and VP'*(uk), the number of
proper words observed in k excerpt which size is N' types. The mathematical
expectation of the proper vocabulary in this excerpt is :
VP'(uk) = VP.u and indicator of
originality :
When this indicator is equal to one, the originality is
average ; above, the excerpt is out of line with the others ;
under, the author used common materials. The proper vocabulary of each
excerpt is always interesting. For example, in General de Gaulle's case,
there are numerous names, indicating application of the same outlines to
new events, other peoples or countries
As for the partition coefficient, accuracy of
computation depends on the number and quality of observations. For example,
no less than ten values are necessary to compute mathematical
expectation.
4. Vocabulary growth and location of thematic
caesura. The partition model also allows estimation, in any
point of a text, of the theoritical number of different words used since
the beginning (under the steady flow hypothesis). The incremental growth in
a part of the text is the ratio of the observed number of different words
to the theoritical value calculated with (2). Thematic caesuras are located
at the points where unusual flows of new words occur. On the contrary, when
this flow is under expected values, a theme is ending or author's
inspiration weakens or, for a politician, he appropriates with difficulty
his vocabulary to a new situation. This model has been applied to speaches
of President Mitterrand (Labbé, 1990a) and General de Gaulle
(Labbé, 1993).
In conclusion, the different formulas we presented there
are easy to compute and the results can be very accurate. Other researches
will be necessary to appreciate interest and limits of our model. In any
case, these calculus set up the problem of textual data quality : if
this quality is poor, the accuracy of calculus is useless. Unfortunatly,
for French language, there are many standards for text encoding and these
standards have a heavy influence on results (see Ménard,
1983 and Salem, 1993).
Table. Press conferences by Général de
Gaulle
("Saint-Cloud" standards for text encoding : Lafon,
1985 ; Labbé, 1988a)
Conferences |
N (types) |
V (words) |
V'(Muller) (5299 words) |
V'(pa) (5299 words) |
P (speciali-sation) |
Diversity (1000 words) |
Originality |
Nov. 10th 1959 |
6311 |
1566 |
1413 |
1413 |
0,00 |
474 |
0,92 |
Sept. 5th 1960 |
6808 |
1580 |
1355 |
1352 |
0,02 |
433 |
0,83 |
Apr. 11th 1961 |
6597 |
1599 |
1391 |
1377 |
0,13 |
418 |
0,89 |
Sept. 5th 1961 |
5299 |
1423 |
1423 |
1423 |
0,02 |
411 |
0,89 |
May 15th 1962 |
5946 |
1434 |
1335 |
1330 |
0,08 |
419 |
0,81 |
Jan. 14th 1963 |
6906 |
1687 |
1429 |
1421 |
0,06 |
443 |
0,84 |
Jul. 29th 1963 |
6682 |
1743 |
1504 |
1504 |
0,00 |
470 |
1,13 |
Jan. 31th 1964 |
7686 |
1974 |
1548 |
1504 |
0,23 |
420 |
1,16 |
Sept. 23th 1964 |
5886 |
1633 |
1528 |
1522 |
0,09 |
459 |
1,10 |
Feb. 4th 1965 |
6543 |
1814 |
1564 |
1564 |
0,00 |
448 |
1,19 |
Sept. 9th 1965 |
7958 |
2023 |
1583 |
1561 |
0,16 |
480 |
1,04 |
Feb. 21th 1966 |
6148 |
1655 |
1506 |
1496 |
0,13 |
448 |
1,10 |
Oct. 28th 1966 |
7446 |
1865 |
1498 |
1456 |
0,24 |
412 |
1,05 |
May 16th 1967 |
6514 |
1786 |
1562 |
1559 |
0,03 |
475 |
1,04 |
Nov. 27th 1967 |
7895 |
2028 |
1562 |
1537 |
0,13 |
446 |
1,37 |
Sept. 9th 1968 |
6027 |
1639 |
1514 |
1514 |
0,00 |
481 |
1,11 |
REFERENCES
Bernet, Ch. (1988), "Faits lexicaux. Richesse du
vocabulaire. Résultats" in D. Labbé, Ph. Thoiron and D.
Serant, Etudes sur la richesse et la structure lexicales,
Paris-Genève, Slatkine-Champion, p 1-12.
Hubert P. and Labbé D. (1988a), "Note sur
l'approximation de la loi hypergéométrique par la formule de
Muller", in D. Labbé, Ph. Thoiron and D. Serant, Etudes sur la
richesse et la structure lexicales, Paris-Genève,
Slatkine-Champion, p 77-91.
Hubert, P. and Labbé, D. (1988b), "Un
modèle de partition du vocabulaire", in D. Labbé, Ph. Thoiron
and D. Serant, Etudes sur la richesse et la structure lexicales,
Paris-Genève, Slatkine-Champion, p 93-114.
Labbé, D. (1990a), Normes de
dépouillement et procédures d'analyse des textes
politiques, Grenoble, CERAT.
Labbé, D. (1990b), Le vocabulaire de
François Mitterrand, Paris, Presses de la FNSP.
Labbé, D. (1993), Un modèle d'analyse
du vocabulaire, Communication aux secondes journées d'analyse de
données textuelles, Montpellier, octobre 1993.
Lafon, P. (1984), Dépouillement et
statistiques en lexicométrie, Paris-Genève,
Slatkine-Champion.
Lafon, P., Lefevre, J. et al. (1985), Le Machinal
(Principes d'enregistrement informatique des textes), Paris,
Klincksieck.
Ménard, N. (1983), Mesure de la richesse
lexicale, Genève-Paris, Slatkine-Champion.
Muller, Ch. (1977), Principes et méthodes de
statistique lexicale, Paris, Hachette.
Muller, Ch. (1979a), "Calcul des probabilités et
calcul d'un vocabulaire" in Langue française et linguistique
quantitative, Genève-Paris, Slatkine-Champion, p 167-176.
Muller, Ch. (1979b), "Sur la mesure de la richesse
lexicale. Théorie et expériences" in Langue
française et linguistique quantitative, Paris-Genève,
Slatkine-Champion, p 281-307.
Muller, Ch. (1985), "Les classes de fréquence" in
Langue française, linguistique quantitative, informatique,
Genève-Paris, Slatkine-Champion, p 53-58.
Salem A. (1993), Méthodes de la statistique
textuelle, Thèse de doctorat d'Etat, Paris, Université
Paris III, 3 tomes.
|